My First Steps
First thing I .ylraldid was just pull up the basic matchup. Okay, Troy, Sun Belt conference. Ole Miss, SEC. Right away, you know there's usually a difference in the level of competition they face week in, week out. Ole Miss playing in the SEC means they bang heads with tough teams regularly.
Digging into Troy
Then I spent some time looking specifically at Troy. Watched some highlights from their recent games, checked their scores. They looked pretty competent, honestly. Seemed like they had a decent defense for their conference level, and their offense could move the ball. But the big question mark was how they'd handle the step up in athlete quality against an SEC team. It's just different speed, different size up front.
Checking Out Ole Miss
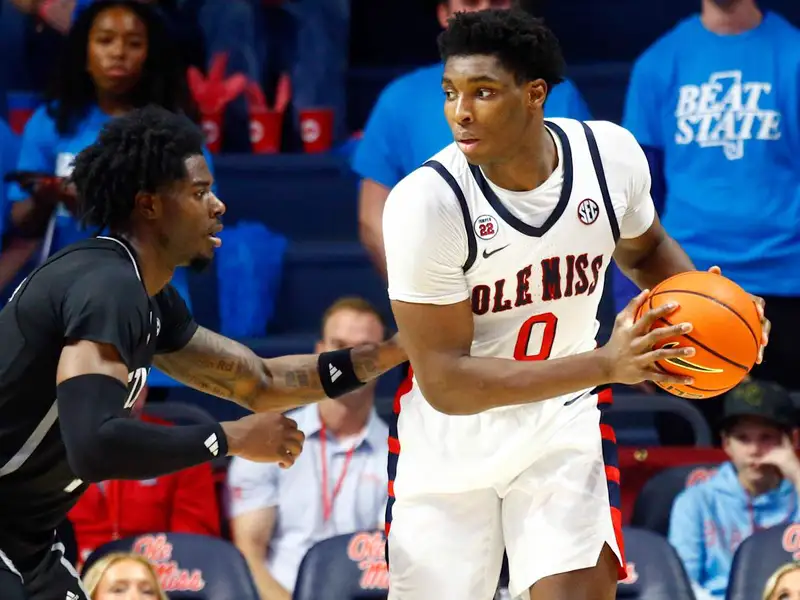
Next, I turned my attention to Ole Miss. Lane Kiffin's teams are usually known for offense, right? So I looked at their points per game, their key offensive players. Yeah, they could definitely score. Saw some games where they just lit up the scoreboard. But then I also looked at their defense. Sometimes seemed like they gave up quite a few points too. It wasn't like looking at Georgia's defense or something. They seemed beatable on that side of the ball.
Putting It Together
So, the main thing I kept coming back to was the Ole Miss offense versus the Troy defense. Could Troy slow down that high-tempo attack enough to keep it close? My gut feeling was... probably not consistently. Maybe for a bit, but Ole Miss has too many weapons. Then I thought about Troy's offense against the Ole Miss defense. Troy might be able to score some points. I didn't think Ole Miss would completely shut them out, especially if Ole Miss's defense was having one of its less-than-stellar days.
I also considered the location. Pretty sure Ole Miss was playing at home. That's always an advantage, the crowd noise, familiarity.
Making the Call
After mulling it all over, I just felt like Ole Miss had too much firepower and the SEC pedigree. Troy's a solid program, definitely one of the better teams in their conference, but that jump to playing an SEC team, especially one with a potent offense like Ole Miss, is tough.
My thinking was Ole Miss would win the game. I figured Troy would put up a fight, maybe cover the spread depending on what it was, but ultimately Ole Miss would pull away. I didn't settle on an exact score prediction, but I leaned towards Ole Miss winning by a couple of touchdowns, maybe more if their offense really clicked.
That was pretty much it. Looked at the teams, considered the conference difference, focused on the offense vs defense matchup, and went with the team that seemed to have the higher ceiling and was playing at home. Just my two cents on how I approached it!