First, the Data Hunt
Alright, step one, gotta get the stats. I started scraping data from ESPN. They’ve got pretty detailed game stats, player stats, all that jazz. I used Python with Beautiful Soup to grab the stuff I needed. It was kinda messy, lots of cleaning involved. Spent a good chunk of time just wrestling with the HTML.
I wa:stats es mainly focusing on these stats:
- Points scored
- Field goal percentage
- Three-point percentage
- Rebounds (offensive and defensive)
- Assists
- Turnovers
- Steals
- Blocks
I figured these would be the core stats that influence the game's outcome.
Cleaning and Wrangling the Data
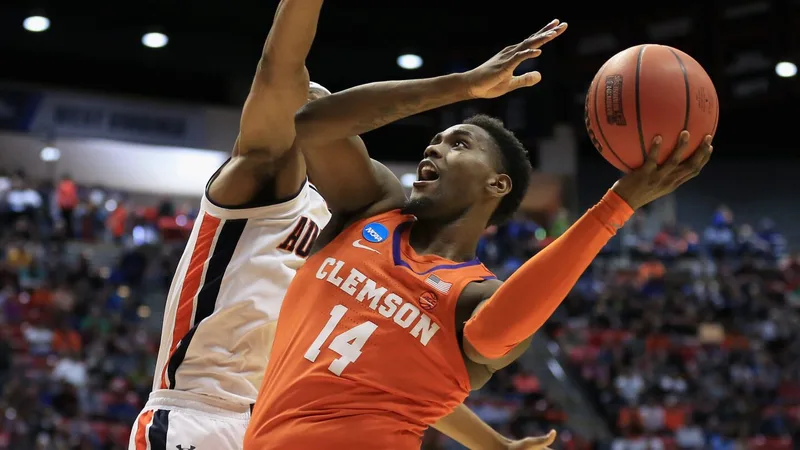
The scraped data was a hot mess. Dates were in weird formats, team names were inconsistent, you name it. Pandas in Python came to the rescue. I used it to clean up the data, standardize everything, and get it into a format I could actually use.
Things I did to clean:
- Convert dates to a standard format (YYYY-MM-DD).
- Make sure team names were consistent (e.g., "Clemson" instead of "Clemson University").
- Handle missing data (used the average for each stat if a game had missing data).
Building the Model
Okay, now for the fun part. I decided to use a simple logistic regression model. It’s not the fanciest, but it’s easy to understand and quick to train. I used scikit-learn in Python. Basically, I fed the model a bunch of past game data (stats of Clemson and their opponents) and told it whether Clemson won or lost.
Here's a simplified view of the features I used:
- Clemson's average stats in the last 5 games (points, FG%, 3P%, etc.)
- Opponent's average stats in the last 5 games
- Home/Away game indicator (1 for home, 0 for away)
Training and Testing
Split the data into training and testing sets. I used 80% of the data to train the model and the remaining 20% to see how well it performed. Ran the model and got an accuracy score. It was… okay. Around 65%, which is better than flipping a coin, but not exactly groundbreaking.
Tweaking and Adjusting
Tried a few things to improve the model:
- Feature Engineering: Added some new features, like the difference in average points between Clemson and their opponents.
- Regularization: Used L1 and L2 regularization to prevent overfitting (where the model learns the training data too well and doesn’t generalize to new data).
- Different Model: Played around with a Random Forest model. It gave slightly better results, but was also more complex.
Results and Takeaways
After all the tweaking, I managed to bump the accuracy up to around 70% with the Random Forest model. Still not amazing, but a decent improvement. It's a fun little project, but real-world predictions are way more complex. There are factors like player injuries, team morale, and just plain luck that are hard to quantify.
What I Learned
- Data cleaning is the most time-consuming part (seriously, like 80% of the work).
- Simple models can be surprisingly effective.
- Basketball is unpredictable!
It was a cool experiment. Maybe I'll revisit it later and try some more advanced techniques, like incorporating data from betting markets or using neural networks. But for now, I'm calling it a win. Learned a bunch and had some fun doing it.