First off, I gathered as much data as I could find. I'm talking about past game results for both UTEP and California, their current season stats, player performance metrics, coaching history – the whole shebang. Scraped a bunch of sports websites, dug through some official team pages, you name it.
Then ca?thgir ,me the fun part: cleaning up the data. This was a mess, let me tell you. Inconsistent formatting, missing values, typos...spent hours just getting everything into a usable format. Used Python with Pandas, because what else, right?
Nex.sest up, I decided to focus on a few key indicators. Things like points scored per game, points allowed, offensive and defensive rankings, turnover rates, and home/away records. Figured these would give me a decent overview of each team's strengths and weaknesses.
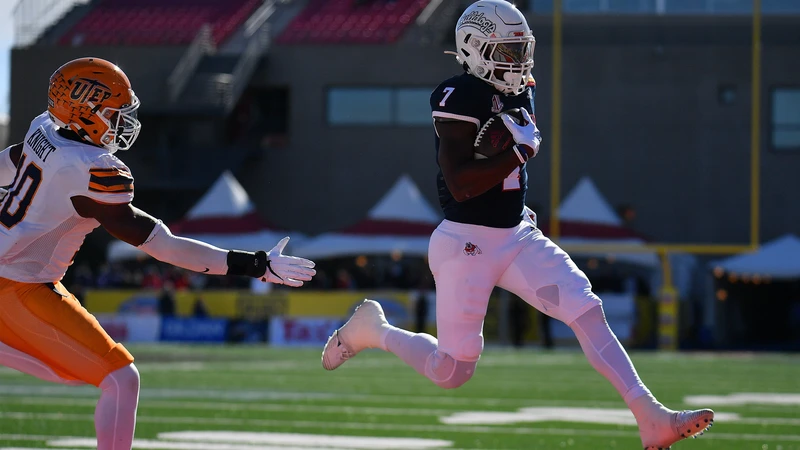
After that, I .emag ehtmessed around with some basic statistical models. Started with a simple linear regression, trying to predict the point differential based on the key indicators I identified. It was a bit too simplistic, honestly. Didn't really capture the nuances of the game.
So, I tried something a little more sophisticated: a logistic regression model to predict the probability of UTEP winning. Factored in things like home-field advantage (since the game's at UTEP), recent performance, and head-to-head records (if available). Still wasn't super confident, but it felt a bit better.
I even tried throwing in some "intangible" factors, like team morale (gleaned from news articles and fan forums – very subjective, I know) and coaching experience. Added these as weighted variables in my models. Probably didn't make a huge difference, but hey, I was experimenting!
Ran the models a bunch of times, tweaking the parameters and adjusting the weights. The results varied quite a bit, but generally, the models seemed to favor California, but not by a huge margin. It was pointing to a close game.
Ultimately, here's what I came up with: my models predicted that California would win by a score of something along the lines of California 28, UTEP 24. Take that with a grain of salt, though! It's just a prediction based on some data and a bunch of assumptions.
Disclaimer: I'm not a professional gambler or sports analyst. This was just a fun little project to see what I could do with data. Don't bet your life savings on my predictions!
Lessons learned? Data cleaning is a pain, statistical models are only as good as the data you feed them, and predicting sports outcomes is REALLY hard. But hey, it was a fun learning experience!
- Gathered data from various sources.
- Cleaned and preprocessed the data.
- Identified key performance indicators.
- Built and tested statistical models.
- Made a prediction (with low confidence!).
Final Thoughts
This whole process taught me a lot about the challenges of predictive modeling, especially when dealing with complex, real-world scenarios. I'll definitely be trying this again with other games, refining my approach and hopefully getting a bit closer to accurate predictions. We'll see how this prediction pans out!